Data analysis serves as the cornerstone of rigorous research, providing scholars with the tools to derive profound insights from meticulously collected data. This article delves into an exploration of various types and methods of data analysis, elucidating their applications and profound significance in the realm of research. In adherence to academic standards, we will examine descriptive analysis, inferential analysis, qualitative analysis, multivariate analysis, and time series analysis, each playing a distinctive role in the analytical arsenal of researchers.
Descriptive Analysis
At the inception of data analysis, researchers engage in descriptive analysis to uncover inherent patterns and trends residing within the dataset. Descriptive statistics, including measures of central tendency such as mean, median, and mode, and measures of dispersion such as standard deviation, provide a comprehensive overview of the data’s characteristics. This method lays the foundation for subsequent analyses, guiding researchers in formulating hypotheses for further exploration. As foundational as it is, descriptive analysis serves as the initial step in navigating the complex landscape of data analysis.
Inferential Analysis
Moving beyond the surface, inferential analysis assumes a pivotal role in drawing meaningful conclusions from a smaller sample of data and generalising them to a larger population. Techniques such as hypothesis testing and regression analysis empower researchers to make predictions and infer relationships between variables. The complexity of inferential analysis necessitates a profound understanding of statistical methods to ensure the validity and reliability of research findings. In this context, scholars are compelled to traverse the intricate terrain of statistical inference, bridging the gap between observed data and broader theoretical implications.
Qualitative Analysis
While quantitative methods dominate many research fields, qualitative analysis contributes an indispensable layer of depth by delving into the intricacies of human experiences and behaviours. Techniques such as content analysis, thematic analysis, and grounded theory facilitate the extraction of rich, context-specific insights from qualitative data. In the context of the social sciences and humanities, where the exploration of human perspectives is paramount, qualitative analysis emerges as an invaluable tool. This section illuminates the nuanced nature of qualitative analysis, highlighting its role in enriching the tapestry of empirical research.
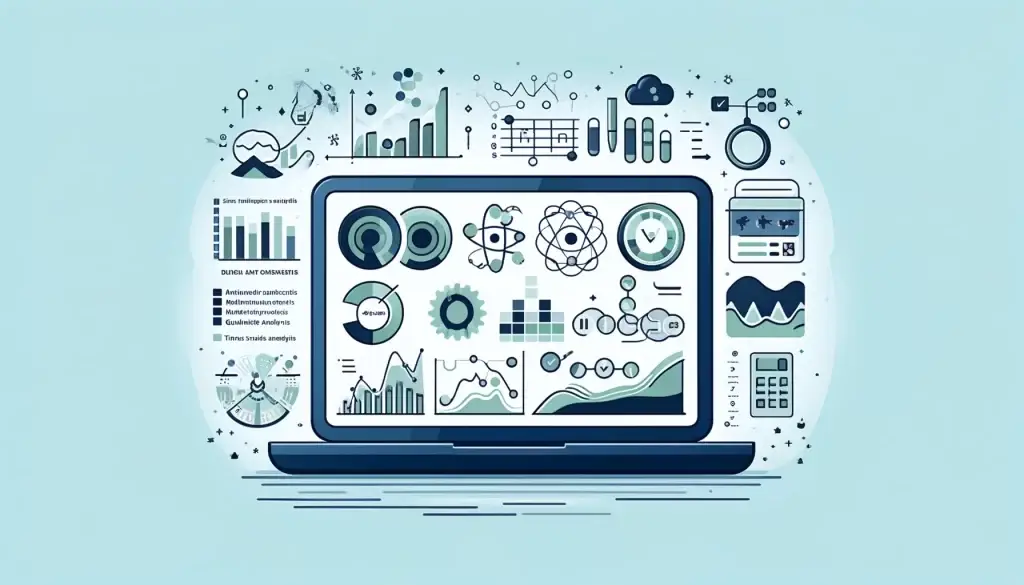
Multivariate Analysis
To capture the complexity inherent in real-world phenomena, researchers frequently turn to multivariate analysis. This approach considers the interactions among multiple variables simultaneously, offering a more comprehensive understanding of the relationships within the data. Methods such as factor analysis and cluster analysis aid in identifying latent structures and grouping variables based on similarities, fostering a deeper exploration of underlying patterns. The intricate interplay between variables in multivariate analysis adds a layer of sophistication to the analytical process, compelling scholars to navigate the intricate landscape of multidimensional data.
Time Series Analysis
In fields where data evolves over time, time series analysis becomes an indispensable tool for researchers. This method allows scholars to identify temporal patterns, trends, and fluctuations within a dataset. Whether studying economic indicators, climate data, or stock prices, time series analysis provides the tools to unravel the temporal dynamics that shape the observed phenomena. This section underscores the temporal dimension inherent in various fields of study, emphasising the significance of time series analysis in extracting meaningful insights from time-varying datasets.
Finally
A nuanced comprehension of various types and methods of data analysis is imperative for researchers seeking to extract profound insights from their datasets. By seamlessly integrating descriptive, inferential, qualitative, multivariate, and time series analyses into their methodological repertoire, scholars can adeptly navigate the multifaceted landscape of research, contributing substantively to the advancement of knowledge in their respective fields.
References
Hair, J. F., Black, W. C., Babin, B. J., & Anderson, R. E. (2018). Multivariate Data Analysis (8th ed.). Cengage Learning.
Creswell, J. W., & Creswell, J. D. (2017). Research Design: Qualitative, Quantitative, and Mixed Methods Approaches (4th ed.). SAGE Publications.
Montgomery, D. C., Peck, E. A., & Vining, G. G. (2012). Introduction to Linear Regression Analysis (5th ed.). Wiley.